Keynote speakers
Prof. Alan C Evans 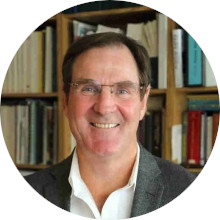
McConnell Brain Imaging Centre, McGill University
Brain Imaging: Past, Present and Future
Biography: Alan Evans is James McGill Professor of Neurology and Psychiatry at McGill. Trained in physics and protein crystallography, his current research covers multi-modal brain imaging and structural network modeling. He is Scientific Director of both McGill’s $84M CFREF project Healthy Brains for Healthy Lives and the Canadian Open Neuroscience Platform. He is also co-Director of the Ludmer Centre for Neuroinformatics and Mental Health, using high-performance computing to integrate imaging, behavior, genetics data in research into neurodegeneration and neurodevelopment. He has 653 peer-reviewed papers (ISI h-index=130 ; Google Scholar h-index=192). In 2014, he received the Vezina Prize for QuébecNeuroradiology, the national Margolese Human Brain Disorders Prize and was a Highly Cited Scientist (149,596 citations ; top 1%) for Neuroscience and Behavior. He is a Fellow of the Royal Society of Canada and 2017 Chair of the Organization for Human Brain Mapping (OHBM). In 2016, he received the Wilder Penfield Prix du Québec. In 2017, he was awarded the Senate of Canada 150 Medal, ranked #6 in its list of ten most influential brain scientists of the modern era by Science magazine and inducted as a Fellow of the College of Academic Health Sciences. In 2018, he was awarded the Heinz Lehmann Prize for services to Neuropsychopharmacology, the Victor Dahdaleh Chair in Neurosciences and the Club de Recherches Clinique du Québec Mentorship Prize. He was awarded the OHBM Glass Brain Award in 2019 and the Killam Prize in Health Sciences in 2020, both for lifetime achievement.
Prof. Nikos Paragios 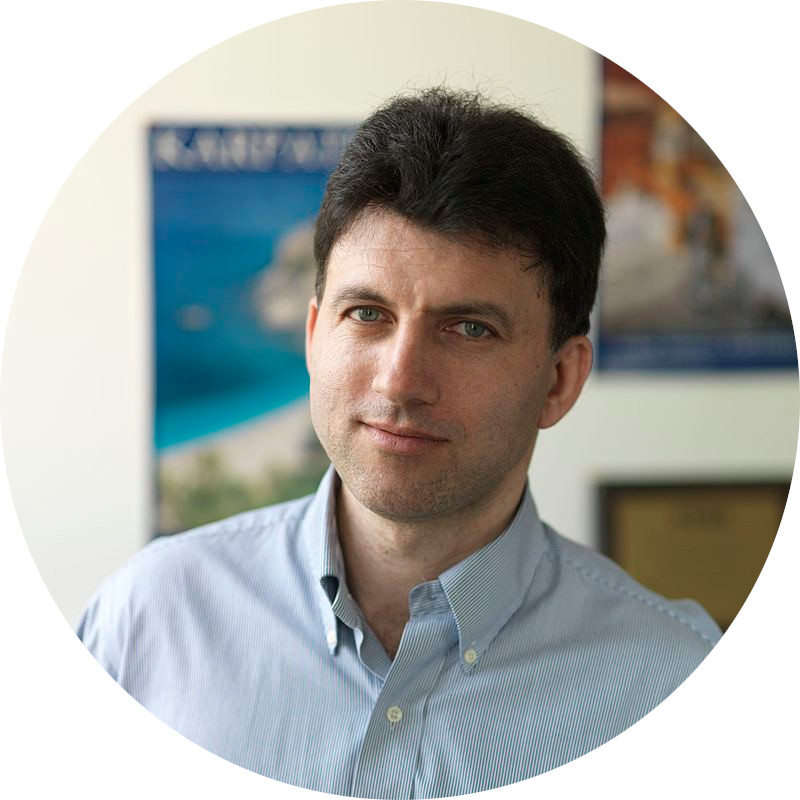
CentraleSupélec
Holistic AI-Driven Quantification, Staging and Prognosis of COVID-19 Pneumonia
Abstract: Improving screening, discovering therapies, developing a vaccine and performing staging and prognosis are decisive steps in addressing the COVID-19 pandemic. Staging and prognosis are especially crucial for organizational anticipation (intensive-care bed availability, patient management planning) and accelerating drug development; through rapid, reproducible and quantified response-to-treatment assessment. In this letter, we report on an artificial intelligence solution for performing automatic staging and prognosis based on imaging, clinical, comorbidities and biological data. This approach relies on automatic computed tomography (CT)-based disease quantification using deep learning, robust data-driven identification of physiologically-inspired COVID19 holistic patient profiling, and strong, reproducible staging/outcome prediction with good generalization properties using an ensemble of consensus methods. Highly promising results on multiple independent external evaluation cohorts along with comparisons with expert human readers demonstrate the potentials of our approach. The developed solution offers perspectives for optimal patient management, given the shortage of intensive care beds and ventilators, along with means to assess patient response to treatment.
Biography: Nikos Paragios (47) is distinguihsed professor of Mathematics (on partial leave) at CentraleSupelec, the school of engineering of the University of Paris Saclay and CEO/CSO of TheraPanacea. Prior to that he was senior fellow (2015-2020) at the Institut Universitaire de France, director (2011-2017) of the Center for Visual Computing at CentraleSupelec, scientific leader (2007-2017) at Inria, professor (2004-2005, 2011-2013) at the Ecole de Ponts ParisTech, and affiliated with Siemens Corporate Research (Princeton, NJ, 1999-2004) as a project manager, senior research scientist and research scientist. N. Paragios was an adjunct professor at Rutgers (2002) and at New York University (2004) and a visiting professor at Yale (2007) and at University of Houston (2009). Professor Paragios is a fellow of IEEE (class of 2011), has co-edited four books, published more than 300 papers in the most prestigious journals and conferences (DBLP server), and holds 30 international patents. His work has approx 25,000 citations according to Google Scholar and his H-number (03/2020) is 68. Professor Paragios is the Editor in Chief of the Computer Vision and Image Understanding Journal (CVIU) since 2013 and serves as member of the editorial board for the Medical Image Analysis Journal (MedIA) while has served in editorial board of numerous and prestigious international journals (IEEE T-PAMI, IJCV, IVC, MVA, JMIV, SIIMS...). Pr. Paragios received an IBM faculty award in 2014, a European Research Council excellence award in 2011 while in 2008 was the laureate of the Greece's (nationality or descent) highest honor (world-wide) for young academics and scientists, the Bodossaki Prize. In 2006, he was named one of the top 35 innovators world-wide innovators under the age of 35 from the MIT's Technology Review. Professor Paragios is member of the scientific council of Safran conglomerate, member of the advisory board of the Data Healthcare Institute and member of the academic research council of the Université Paris Descartes.
Dr. Elsa Angelini 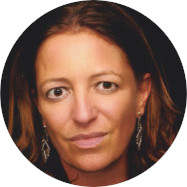
Institute for Translational Medicine and Therapeutics (ITMAT), Imperial College London
Unsupervised Learning and Domain Adaptation for Subtyping of Emphysema on Very Large Cohorts of Lung CT Scans
Biography: Elsa D. Angelini received the B.Sc. degree in engineering from Ecole Centrale de Nantes (France), and the MSc and PhD degrees in Biomedical Engineering from Columbia University (NY, USA). She joined the Department of Image-Data-Signal at Telecom ParisTech (France) in 2004 where she was an associate Professor until 2011. In 2010, she spent a sabbatical in the CSIRO-Australian e-Health Research Center (Australia). In 2011, she was appointed as a Senior Research Scientist and co-director of the Heffner Biomedical Imaging Laboratory in the Department of Biomedical Engineering at Columbia University (USA). In 2016, she joined Imperial College London (UK) where she is the co-lead of the newly created Data Science Group within the Institute of Translational Medicine and Therapeutics (ITMAT), which is part of the NIHR Imperial Biomedical Research Centre. She has co-authored over 140 publications in the field of bio and medical image analysis. As a Senior IEEE member, she has served multiple roles for the EMB society, as chair of the TMI and ISBI steering committees, Vice-President for the Technical Activities (2017-19), and chair of the Biomedical Imaging and Image Processing (BIIP) Technical Committee (2013-15). She has served as an Associate Editor (AE) for IEEE TBME and JBHI, is a regular AE for ISBI, and PC member for MICCAI. She was the general chair of the ISBI'15 conference, and co-chair (2016-19) of the SPIE Medical-Imaging sub-Conference on Image Processing. Her research interests focus on bio and medical image computing for multi-dimensional imaging modalities, along with machine-learning on imaging, electronic health records, and omics data for patient stratification, outcome prediction, and disease scoring and subtyping.
Prof. Daphne Koller 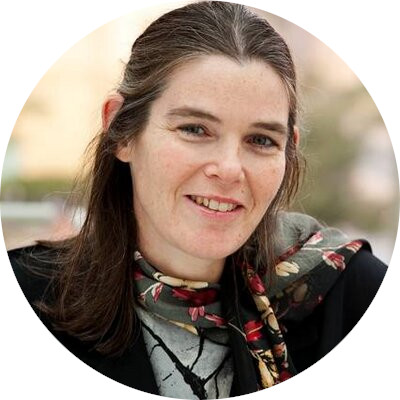
Stanford University
Machine learning: a new approach to drug discovery
Abstract: Modern medicine has given us effective tools to treat some of the most significant and burdensome diseases. At the same time, it is becoming consistently more challenging to develop new therapeutics: clinical trial success rates hover around the mid-single-digit range; the pre-tax R&D cost to develop a new drug (once failures are incorporated) is estimated to be greater than $2.5B; and the rate of return on drug development investment has been decreasing linearly year by year, and some analyses estimate that it will hit 0% before 2020. A key contributor to this trend is that the drug development process involves multiple steps, each of which involves a complex and protracted experiment that often fails. We believe that, for many of these phases, it is possible to develop machine learning models to help predict the outcome of these experiments, and that those models, while inevitably imperfect, can outperform predictions based on traditional heuristics. The key will be to train powerful ML techniques on sufficient amounts of high-quality, relevant data. To achieve this goal, we are bringing together cutting edge methods in functional genomics and lab automation to build a bio-data factory that can produce relevant biological data at scale, allowing us to create large, high-quality datasets that enable the development of novel ML models. Our first goal is to engineer in vitro models of human disease that, via the use of appropriate ML models, are able to provide good predictions regarding the effect of interventions on human clinical phenotypes. Our ultimate goal is to develop a new approach to drug development that uses high-quality data and ML models to design novel, safe, and effective therapies that help more people, faster, and at a lower cost.
Biography: Daphne Koller is CEO and Founder of insitro, a data-driven drug discovery and development company using machine learning and high-throughput biology to transform the way drugs are discovered and delivered to patients. She was also the co-founder, Co-CEO, and President of Coursera, the largest platform for massive open online courses (MOOCs). Daphne was the Rajeev Motwani Professor of Computer Science at Stanford University, where she served on the faculty for 18 years. She has also been Chief Computing Officer of Calico, an Alphabet company in the healthcare space. She is the author of over 200-refereed publications appearing in venues such as Science, Cell, and Nature Genetics. Daphne was recognized as one of TIME Magazine’s 100 most influential people in 2012 and Newsweek’s 10 most important people in 2010. She has been honored with multiple awards and fellowships during her career including the Sloan Foundation Faculty Fellowship in 1996, the ONR Young Investigator Award in 1998, the Presidential Early Career Award for Scientists and Engineers (PECASE) in 1999, the IJCAI Computers and Thought Award in 2001, the MacArthur Foundation Fellowship in 2004, and the ACM Prize in Computing in 2008. Daphne was inducted into the National Academy of Engineering in 2011 and elected a fellow of the American Academy of Arts and Sciences in 2014 and of the International Society of Computational Biology in 2017. Her teaching was recognized via the Stanford Medal for Excellence in Fostering Undergraduate Research, and as a Bass University Fellow in Undergraduate Education.